在实践中,给定一组相同的查询、键和值,我们可能希望我们的模型结合来自同一注意机制的不同行为的知识,例如捕获各种范围的依赖关系(例如,较短范围与较长范围)在一个序列中。因此,这可能是有益的
允许我们的注意力机制联合使用查询、键和值的不同表示子空间。
为此,可以使用以下方式转换查询、键和值,而不是执行单个注意力池h独立学习线性投影。那么这些h投影查询、键和值被并行输入注意力池。到底,h 注意池的输出与另一个学习的线性投影连接并转换以产生最终输出。这种设计称为多头注意力,其中每个hattention pooling outputs 是一个头 (Vaswani et al. , 2017)。使用全连接层执行可学习的线性变换,图 11.5.1描述了多头注意力。
图 11.5.1多头注意力,其中多个头连接起来然后进行线性变换。
import math import torch from torch import nn from d2l import torch as d2l
import math from mxnet import autograd, np, npx from mxnet.gluon import nn from d2l import mxnet as d2l npx.set_np()
import jax from flax import linen as nn from jax import numpy as jnp from d2l import jax as d2l
No GPU/TPU found, falling back to CPU. (Set TF_CPP_MIN_LOG_LEVEL=0 and rerun for more info.)
import tensorflow as tf from d2l import tensorflow as d2l
11.5.1。模型
在提供多头注意力的实现之前,让我们从数学上形式化这个模型。给定一个查询 q∈Rdq, 关键 k∈Rdk和一个值 v∈Rdv, 每个注意力头 hi(i=1,…,h) 被计算为
(11.5.1)hi=f(Wi(q)q,Wi(k)k,Wi(v)v)∈Rpv,
其中可学习参数 Wi(q)∈Rpq×dq, Wi(k)∈Rpk×dk和 Wi(v)∈Rpv×dv, 和f是注意力集中,例如11.3 节中的附加注意力和缩放点积注意力。多头注意力输出是另一种通过可学习参数进行的线性变换Wo∈Rpo×hpv的串联h负责人:
(11.5.2)Wo[h1⋮hh]∈Rpo.
基于这种设计,每个头可能会关注输入的不同部分。可以表达比简单加权平均更复杂的函数。
11.5.2。执行
在我们的实现中,我们为多头注意力的每个头选择缩放的点积注意力。为了避免计算成本和参数化成本的显着增长,我们设置 pq=pk=pv=po/h. 注意h如果我们将查询、键和值的线性变换的输出数量设置为 pqh=pkh=pvh=po. 在下面的实现中, po通过参数指定num_hiddens。
class MultiHeadAttention(d2l.Module): #@save """Multi-head attention.""" def __init__(self, num_hiddens, num_heads, dropout, bias=False, **kwargs): super().__init__() self.num_heads = num_heads self.attention = d2l.DotProductAttention(dropout) self.W_q = nn.LazyLinear(num_hiddens, bias=bias) self.W_k = nn.LazyLinear(num_hiddens, bias=bias) self.W_v = nn.LazyLinear(num_hiddens, bias=bias) self.W_o = nn.LazyLinear(num_hiddens, bias=bias) def forward(self, queries, keys, values, valid_lens): # Shape of queries, keys, or values: # (batch_size, no. of queries or key-value pairs, num_hiddens) # Shape of valid_lens: (batch_size,) or (batch_size, no. of queries) # After transposing, shape of output queries, keys, or values: # (batch_size * num_heads, no. of queries or key-value pairs, # num_hiddens / num_heads) queries = self.transpose_qkv(self.W_q(queries)) keys = self.transpose_qkv(self.W_k(keys)) values = self.transpose_qkv(self.W_v(values)) if valid_lens is not None: # On axis 0, copy the first item (scalar or vector) for num_heads # times, then copy the next item, and so on valid_lens = torch.repeat_interleave( valid_lens, repeats=self.num_heads, dim=0) # Shape of output: (batch_size * num_heads, no. of queries, # num_hiddens / num_heads) output = self.attention(queries, keys, values, valid_lens) # Shape of output_concat: (batch_size, no. of queries, num_hiddens) output_concat = self.transpose_output(output) return self.W_o(output_concat)
class MultiHeadAttention(d2l.Module): #@save """Multi-head attention.""" def __init__(self, num_hiddens, num_heads, dropout, use_bias=False, **kwargs): super().__init__() self.num_heads = num_heads self.attention = d2l.DotProductAttention(dropout) self.W_q = nn.Dense(num_hiddens, use_bias=use_bias, flatten=False) self.W_k = nn.Dense(num_hiddens, use_bias=use_bias, flatten=False) self.W_v = nn.Dense(num_hiddens, use_bias=use_bias, flatten=False) self.W_o = nn.Dense(num_hiddens, use_bias=use_bias, flatten=False) def forward(self, queries, keys, values, valid_lens): # Shape of queries, keys, or values: # (batch_size, no. of queries or key-value pairs, num_hiddens) # Shape of valid_lens: (batch_size,) or (batch_size, no. of queries) # After transposing, shape of output queries, keys, or values: # (batch_size * num_heads, no. of queries or key-value pairs, # num_hiddens / num_heads) queries = self.transpose_qkv(self.W_q(queries)) keys = self.transpose_qkv(self.W_k(keys)) values = self.transpose_qkv(self.W_v(values)) if valid_lens is not None: # On axis 0, copy the first item (scalar or vector) for num_heads # times, then copy the next item, and so on valid_lens = valid_lens.repeat(self.num_heads, axis=0) # Shape of output: (batch_size * num_heads, no. of queries, # num_hiddens / num_heads) output = self.attention(queries, keys, values, valid_lens) # Shape of output_concat: (batch_size, no. of queries, num_hiddens) output_concat = self.transpose_output(output) return self.W_o(output_concat)
class MultiHeadAttention(nn.Module): #@save num_hiddens: int num_heads: int dropout: float bias: bool = False def setup(self): self.attention = d2l.DotProductAttention(self.dropout) self.W_q = nn.Dense(self.num_hiddens, use_bias=self.bias) self.W_k = nn.Dense(self.num_hiddens, use_bias=self.bias) self.W_v = nn.Dense(self.num_hiddens, use_bias=self.bias) self.W_o = nn.Dense(self.num_hiddens, use_bias=self.bias) @nn.compact def __call__(self, queries, keys, values, valid_lens, training=False): # Shape of queries, keys, or values: # (batch_size, no. of queries or key-value pairs, num_hiddens) # Shape of valid_lens: (batch_size,) or (batch_size, no. of queries) # After transposing, shape of output queries, keys, or values: # (batch_size * num_heads, no. of queries or key-value pairs, # num_hiddens / num_heads) queries = self.transpose_qkv(self.W_q(queries)) keys = self.transpose_qkv(self.W_k(keys)) values = self.transpose_qkv(self.W_v(values)) if valid_lens is not None: # On axis 0, copy the first item (scalar or vector) for num_heads # times, then copy the next item, and so on valid_lens = jnp.repeat(valid_lens, self.num_heads, axis=0) # Shape of output: (batch_size * num_heads, no. of queries, # num_hiddens / num_heads) output, attention_weights = self.attention( queries, keys, values, valid_lens, training=training) # Shape of output_concat: (batch_size, no. of queries, num_hiddens) output_concat = self.transpose_output(output) return self.W_o(output_concat), attention_weights
class MultiHeadAttention(d2l.Module): #@save """Multi-head attention.""" def __init__(self, key_size, query_size, value_size, num_hiddens, num_heads, dropout, bias=False, **kwargs): super().__init__() self.num_heads = num_heads self.attention = d2l.DotProductAttention(dropout) self.W_q = tf.keras.layers.Dense(num_hiddens, use_bias=bias) self.W_k = tf.keras.layers.Dense(num_hiddens, use_bias=bias) self.W_v = tf.keras.layers.Dense(num_hiddens, use_bias=bias) self.W_o = tf.keras.layers.Dense(num_hiddens, use_bias=bias) def call(self, queries, keys, values, valid_lens, **kwargs): # Shape of queries, keys, or values: # (batch_size, no. of queries or key-value pairs, num_hiddens) # Shape of valid_lens: (batch_size,) or (batch_size, no. of queries) # After transposing, shape of output queries, keys, or values: # (batch_size * num_heads, no. of queries or key-value pairs, # num_hiddens / num_heads) queries = self.transpose_qkv(self.W_q(queries)) keys = self.transpose_qkv(self.W_k(keys)) values = self.transpose_qkv(self.W_v(values)) if valid_lens is not None: # On axis 0, copy the first item (scalar or vector) for num_heads # times, then copy the next item, and so on valid_lens = tf.repeat(valid_lens, repeats=self.num_heads, axis=0) # Shape of output: (batch_size * num_heads, no. of queries, # num_hiddens / num_heads) output = self.attention(queries, keys, values, valid_lens, **kwargs) # Shape of output_concat: (batch_size, no. of queries, num_hiddens) output_concat = self.transpose_output(output) return self.W_o(output_concat)
为了允许多个头的并行计算,上面的 MultiHeadAttention类使用了下面定义的两种转置方法。具体地,该transpose_output方法将方法的操作反转transpose_qkv。
@d2l.add_to_class(MultiHeadAttention) #@save def transpose_qkv(self, X): """Transposition for parallel computation of multiple attention heads.""" # Shape of input X: (batch_size, no. of queries or key-value pairs, # num_hiddens). Shape of output X: (batch_size, no. of queries or # key-value pairs, num_heads, num_hiddens / num_heads) X = X.reshape(X.shape[0], X.shape[1], self.num_heads, -1) # Shape of output X: (batch_size, num_heads, no. of queries or key-value # pairs, num_hiddens / num_heads) X = X.permute(0, 2, 1, 3) # Shape of output: (batch_size * num_heads, no. of queries or key-value # pairs, num_hiddens / num_heads) return X.reshape(-1, X.shape[2], X.shape[3]) @d2l.add_to_class(MultiHeadAttention) #@save def transpose_output(self, X): """Reverse the operation of transpose_qkv.""" X = X.reshape(-1, self.num_heads, X.shape[1], X.shape[2]) X = X.permute(0, 2, 1, 3) return X.reshape(X.shape[0], X.shape[1], -1)
@d2l.add_to_class(MultiHeadAttention) #@save def transpose_qkv(self, X): """Transposition for parallel computation of multiple attention heads.""" # Shape of input X: (batch_size, no. of queries or key-value pairs, # num_hiddens). Shape of output X: (batch_size, no. of queries or # key-value pairs, num_heads, num_hiddens / num_heads) X = X.reshape(X.shape[0], X.shape[1], self.num_heads, -1) # Shape of output X: (batch_size, num_heads, no. of queries or key-value # pairs, num_hiddens / num_heads) X = X.transpose(0, 2, 1, 3) # Shape of output: (batch_size * num_heads, no. of queries or key-value # pairs, num_hiddens / num_heads) return X.reshape(-1, X.shape[2], X.shape[3]) @d2l.add_to_class(MultiHeadAttention) #@save def transpose_output(self, X): """Reverse the operation of transpose_qkv.""" X = X.reshape(-1, self.num_heads, X.shape[1], X.shape[2]) X = X.transpose(0, 2, 1, 3) return X.reshape(X.shape[0], X.shape[1], -1)
@d2l.add_to_class(MultiHeadAttention) #@save def transpose_qkv(self, X): """Transposition for parallel computation of multiple attention heads.""" # Shape of input X: (batch_size, no. of queries or key-value pairs, # num_hiddens). Shape of output X: (batch_size, no. of queries or # key-value pairs, num_heads, num_hiddens / num_heads) X = X.reshape((X.shape[0], X.shape[1], self.num_heads, -1)) # Shape of output X: (batch_size, num_heads, no. of queries or key-value # pairs, num_hiddens / num_heads) X = jnp.transpose(X, (0, 2, 1, 3)) # Shape of output: (batch_size * num_heads, no. of queries or key-value # pairs, num_hiddens / num_heads) return X.reshape((-1, X.shape[2], X.shape[3])) @d2l.add_to_class(MultiHeadAttention) #@save def transpose_output(self, X): """Reverse the operation of transpose_qkv.""" X = X.reshape((-1, self.num_heads, X.shape[1], X.shape[2])) X = jnp.transpose(X, (0, 2, 1, 3)) return X.reshape((X.shape[0], X.shape[1], -1))
@d2l.add_to_class(MultiHeadAttention) #@save def transpose_qkv(self, X): """Transposition for parallel computation of multiple attention heads.""" # Shape of input X: (batch_size, no. of queries or key-value pairs, # num_hiddens). Shape of output X: (batch_size, no. of queries or # key-value pairs, num_heads, num_hiddens / num_heads) X = tf.reshape(X, shape=(X.shape[0], X.shape[1], self.num_heads, -1)) # Shape of output X: (batch_size, num_heads, no. of queries or key-value # pairs, num_hiddens / num_heads) X = tf.transpose(X, perm=(0, 2, 1, 3)) # Shape of output: (batch_size * num_heads, no. of queries or key-value # pairs, num_hiddens / num_heads) return tf.reshape(X, shape=(-1, X.shape[2], X.shape[3])) @d2l.add_to_class(MultiHeadAttention) #@save def transpose_output(self, X): """Reverse the operation of transpose_qkv.""" X = tf.reshape(X, shape=(-1, self.num_heads, X.shape[1], X.shape[2])) X = tf.transpose(X, perm=(0, 2, 1, 3)) return tf.reshape(X, shape=(X.shape[0], X.shape[1], -1))
让我们MultiHeadAttention使用一个玩具示例来测试我们实现的类,其中键和值相同。因此,多头注意力输出的形状为 ( batch_size, num_queries, num_hiddens)。
num_hiddens, num_heads = 100, 5 attention = MultiHeadAttention(num_hiddens, num_heads, 0.5) batch_size, num_queries, num_kvpairs = 2, 4, 6 valid_lens = torch.tensor([3, 2]) X = torch.ones((batch_size, num_queries, num_hiddens)) Y = torch.ones((batch_size, num_kvpairs, num_hiddens)) d2l.check_shape(attention(X, Y, Y, valid_lens), (batch_size, num_queries, num_hiddens))
num_hiddens, num_heads = 100, 5 attention = MultiHeadAttention(num_hiddens, num_heads, 0.5) attention.initialize() batch_size, num_queries, num_kvpairs = 2, 4, 6 valid_lens = np.array([3, 2]) X = np.ones((batch_size, num_queries, num_hiddens)) Y = np.ones((batch_size, num_kvpairs, num_hiddens)) d2l.check_shape(attention(X, Y, Y, valid_lens), (batch_size, num_queries, num_hiddens))
num_hiddens, num_heads = 100, 5 attention = MultiHeadAttention(num_hiddens, num_heads, 0.5) batch_size, num_queries, num_kvpairs = 2, 4, 6 valid_lens = jnp.array([3, 2]) X = jnp.ones((batch_size, num_queries, num_hiddens)) Y = jnp.ones((batch_size, num_kvpairs, num_hiddens)) d2l.check_shape(attention.init_with_output(d2l.get_key(), X, Y, Y, valid_lens, training=False)[0][0], (batch_size, num_queries, num_hiddens))
num_hiddens, num_heads = 100, 5 attention = MultiHeadAttention(num_hiddens, num_hiddens, num_hiddens, num_hiddens, num_heads, 0.5) batch_size, num_queries, num_kvpairs = 2, 4, 6 valid_lens = tf.constant([3, 2]) X = tf.ones((batch_size, num_queries, num_hiddens)) Y = tf.ones((batch_size, num_kvpairs, num_hiddens)) d2l.check_shape(attention(X, Y, Y, valid_lens, training=False), (batch_size, num_queries, num_hiddens))
11.5.3。概括
多头注意力通过查询、键和值的不同表示子空间结合相同注意力池的知识。要并行计算多头注意的多个头,需要适当的张量操作。
11.5.4。练习
可视化本实验中多个头的注意力权重。
假设我们有一个基于多头注意力的训练模型,我们想要修剪最不重要的注意力头以提高预测速度。我们如何设计实验来衡量注意力头的重要性?
-
pytorch
+关注
关注
2文章
762浏览量
12836
发布评论请先 登录
相关推荐
基于SLH89F5162的左额电压信号的注意力比拼
急:基于Labview技术的驾驶员注意力监测系统的基本思路
抬头显示(HUD)让驾驶者注意力更集中
基于labview的注意力分配实验设计
深度分析NLP中的注意力机制
融合双层多头自注意力与CNN的回归模型
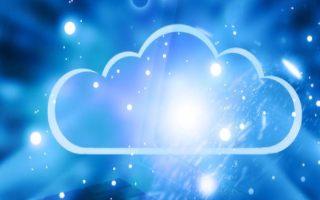
评论